Understanding the Importance of Training Data for Self Driving Cars
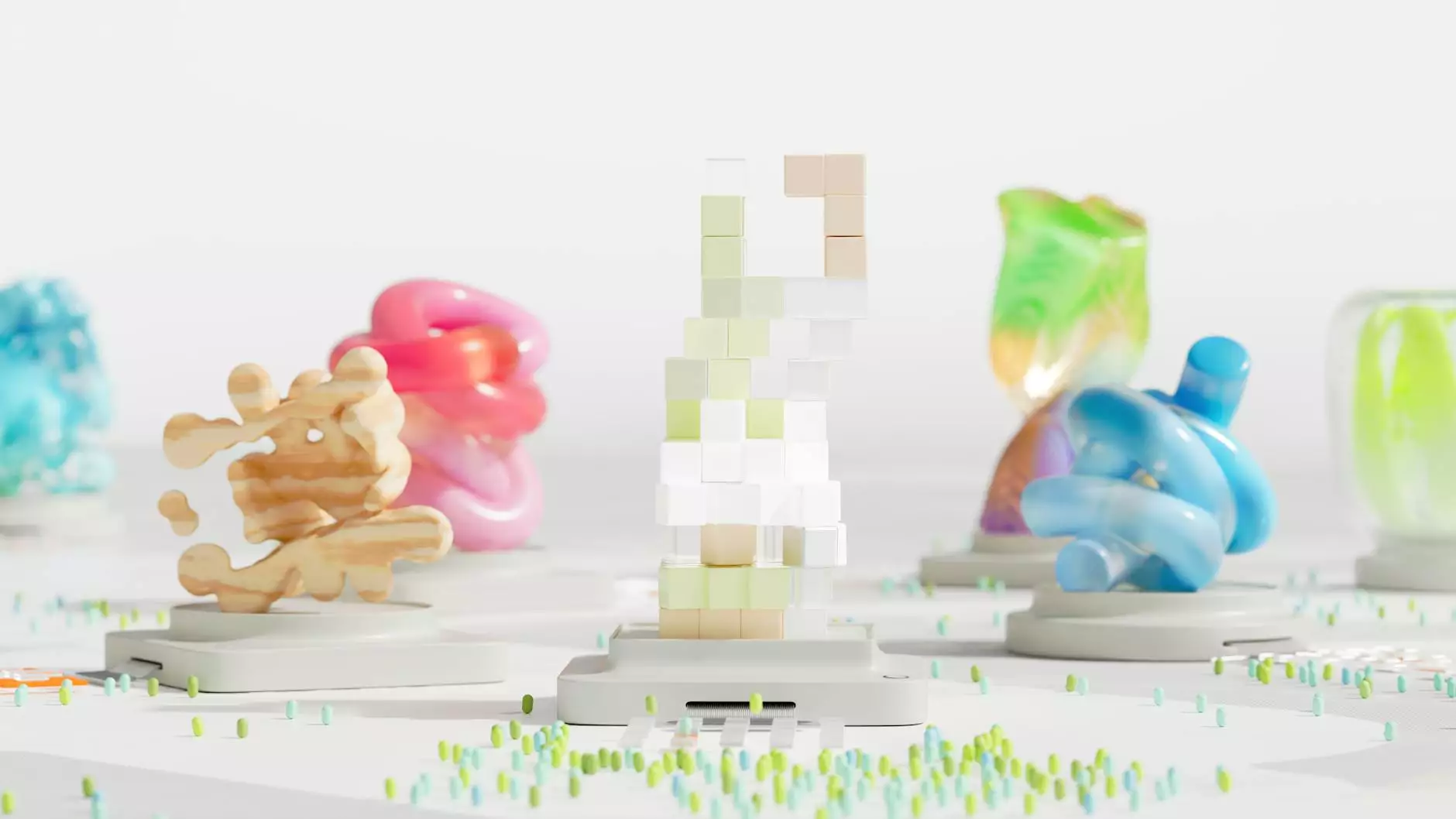
The automotive industry is undergoing a monumental revolution with the advent of self-driving cars. As technology continues to evolve, one crucial element stands out in ensuring the safety and efficiency of these autonomous vehicles: training data for self driving cars. This article delves deep into what training data is, why it is essential, and how it shapes the future of driving.
What is Training Data?
Training data refers to the vast amounts of information collected and utilized by machine learning algorithms to teach self-driving cars how to perceive their environment. This data encompasses various types of information that help algorithms make real-time decisions.
- Sensor Data: Information collected from sensors such as LIDAR, cameras, and radar.
- Environmental Data: Details about different driving conditions, including weather, traffic patterns, and road types.
- User Behavior Data: Insights into how human drivers react in various scenarios.
- Location Data: GPS data that helps cars understand their position in the world.
Why is Training Data Crucial for Self Driving Cars?
The necessity of training data for self driving cars cannot be overstated. Here are several reasons highlighting its significance:
1. Enhances Perception Algorithms
Training data enables perception algorithms to identify and classify objects accurately. For example, a car must distinguish between pedestrians, cyclists, and other vehicles. Robust datasets improve the car’s capability to understand complex scenarios, making it safer on the road.
2. Reduces Accidents
Autonomous vehicles trained with extensive datasets have the potential to reduce the number of accidents significantly. By learning from a multitude of driving scenarios, these vehicles can predict and react to hazardous situations more efficiently, minimizing the risk of collisions.
3. Improves Decision-Making
Decision-making is a critical aspect of driving. Comprehensive training data allows algorithms to learn the best maneuvers in various situations, whether it involves merging into traffic, navigating roundabouts, or responding to sudden obstacles.
4. Adapts to Different Environments
Self-driving cars operate in diverse environments, from bustling urban centers to quiet rural roads. Training data tailored to these varied settings ensures that vehicles can adapt their driving styles and strategies accordingly.
Types of Training Data Used in Self Driving Cars
To build algorithms capable of steering a vehicle safely, manufacturers gather various types of training data:
1. Visual Data
Visual data is primarily collected through cameras mounted on the vehicle. This data is crucial for object detection and recognition. It helps self-driving cars understand traffic signs, lane markings, and the surrounding environment.
2. LIDAR Data
LIDAR technology provides a detailed, three-dimensional view of the environment. It creates precise maps of the surroundings, giving self-driving cars crucial information about objects’ distances and shapes.
3. Radar Data
Radar systems are used to detect the speed and location of objects around the car. This data is particularly useful in adverse weather conditions where visual data may be compromised.
4. Simulated Data
Simulated environments allow companies to create vast amounts of training data without the risks associated with real-world testing. These simulations can represent countless driving scenarios that might be difficult to encounter in reality.
Gathering and Processing Training Data
The process of gathering and processing training data is intricate and multifaceted. Here are the key steps involved:
1. Data Collection
The first step is extensive data collection from various sources, including on-road testing, simulations, and existing datasets from third-party providers. Data from real-world driving provides practical insights that are invaluable.
2. Data Annotation
Once collected, the data must be meticulously annotated. This involves labeling objects within images, tagging data points, and ensuring that all relevant information is categorized correctly. Annotation is a labor-intensive process but is critical for effective training.
3. Data Augmentation
Data augmentation techniques can artificially expand datasets. For instance, techniques such as flipping, rotating, or changing the lighting of images help create variations that improve the robustness of the training process.
4. Continuous Learning
Self-driving systems must continuously learn and adapt. As new data is gathered from operations in real-world conditions, it is fed back into the training system to refine algorithms further, ensuring they evolve with changing environments and driving behaviors.
Challenges in Training Data for Self Driving Cars
While the potential of training data for self driving cars is immense, several challenges still persist:
1. Data Diversity
One of the primary challenges is ensuring data diversity. A model trained on a narrow dataset may fail in real-world situations where conditions differ significantly. Comprehensive data from various environments, traffic conditions, and weather scenarios is essential.
2. Quality of Data
High-quality, accurate, and consistent data is crucial. Poor-quality data can lead to faulty models, resulting in unreliable vehicle behavior. Therefore, data validation and quality control are paramount.
3. Privacy Concerns
The collection of data, especially that involving individuals, raises privacy concerns. Mitigating these concerns while gathering necessary data is a delicate balance that companies must navigate.
4. Computational Resources
Processing large datasets demands significant computational power. Companies must invest in advanced hardware and software to manage and analyze data efficiently.
The Future of Training Data in Self Driving Cars
The future of training data for self driving cars is promising and full of potential innovations. Some key trends and developments to watch include:
1. Increased Use of Artificial Intelligence
The incorporation of artificial intelligence in data processing will enhance the speed and efficiency of training algorithms. AI can help in rapid identification of patterns and trends in data that human analysts might overlook.
2. Real-Time Data Processing
Future self-driving cars will likely process data in real time to react instantaneously to changing environments. This requires robust infrastructure and advanced data processing capabilities to ensure safety and reliability.
3. Collaboration and Data Sharing
Collaborations between companies may lead to shared datasets that enhance the safety and effectiveness of self-driving systems. By pooling resources, companies can address data diversity challenges more effectively.
4. Regulatory Developments
As the technology advances, regulations surrounding data collection, privacy, and vehicle operations will evolve. Businesses must stay informed and compliant to ensure their technologies are not only innovative but also legally sound.
Conclusion
In conclusion, training data for self driving cars is the backbone of the autonomous vehicle industry. It influences every aspect of vehicle intelligence, from perception to decision-making. As technology advances, the methods of gathering, processing, and utilizing this data will continue to evolve, paving the way for safer and more reliable self-driving solutions. For companies involved in software development, particularly in autonomous driving technology, investing in high-quality training data is essential for future success. Embracing the challenges and opportunities in this field will not only drive innovation but also reshape the future of transportation as we know it.